Dismiss Notice
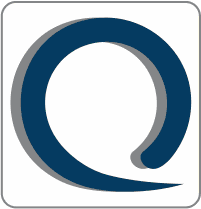
You must be a registered member in order to post messages and view/download attached files in this forum.
Click here to register.
Click here to register.
CCF Design Axial Points Outliers
Discussion in 'DOE - Design of Experiments' started by talez2810, Nov 9, 2017.