Dismiss Notice
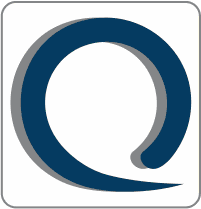
You must be a registered member in order to post messages and view/download attached files in this forum.
Click here to register.
Click here to register.
Capability and Parallel Processes
Discussion in 'Capability - Process, Machine, Gage …' started by ncwalker, Jun 8, 2018.