Dismiss Notice
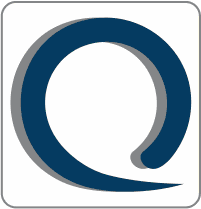
You must be a registered member in order to post messages and view/download attached files in this forum.
Click here to register.
Click here to register.
Which tool to use?
Discussion in '5S, 5Why, 8D, TRIZ, SIPOC, RCA, Shainin Methods...' started by Colin Pitman, Sep 11, 2020.