Dismiss Notice
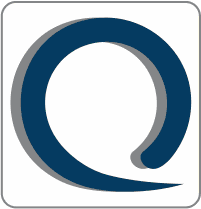
You must be a registered member in order to post messages and view/download attached files in this forum.
Click here to register.
Click here to register.
SPC A-D TEST FAIL
Discussion in 'SPC - Statistical Process Control' started by Sunil, Jan 1, 2016.